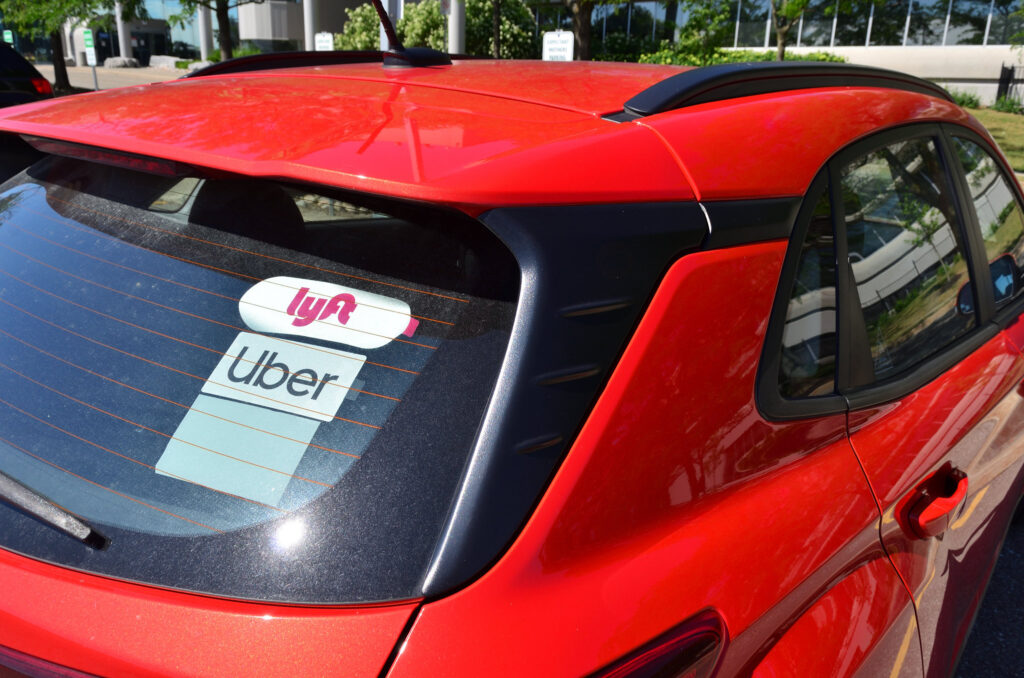
The panoptic mode of automation as seen through Uber and Lyft
Article by Lungani Hlongwa.
Abstract: Many Uber and Lyft drivers are aware that they are constantly being monitored by their passengers or through their mobile devices while on the job. According to Jeremy Bentham and Michel Foucault’s notion of panopticism, the awareness of being monitored shapes one’s conduct to the benefit of the invisible watcher, which, in this case, is the platform owner. Using Uber and Lyft as combined case studies, this article discusses what the author refers to as the ‘panoptic mode of automation’ – the operationalization of surveillance to promote desirable behavior on the job, which is then ‘datafied’ and used to build automated systems. To support this argument, the author employs critical patent analysis to analyze some of the techniques used by Uber and Lyft to engender panoptic power onto their platforms. In our age of ubiquitous computing, perhaps the concept of panopticism is more relevant than when it was first introduced.
Keywords: Uber, Lyft, patents, panopticism, on-demand platform
Header Image “Uber Lyft Car” by “Open Grid Scheduler / Grid Engine” is licensed under CC BY-NC-ND
Introduction
On-demand platforms are known for their voracious appetite for data. Such data is obtained through various means of digital surveillance and is key to the service offerings of on-demand platforms (Sadowski, 2019). For example, Uber and Lyft gather data on drivers to match them with ride requestors. However, driver data may sometimes be used for reasons other than providing services. One such use is building machine learning algorithms to drive autonomous vehicles (Korosec, 2020). Uber and Lyft have been on the receiving end of the growing ‘techlash’ against platform surveillance. In various online forums and interviews, drivers for these platforms have expressed concerns of being constantly monitored by the apps on their mobile devices and their passengers (Rosenblat & Stark, 2016). On both platforms, ride-takers are requested to give performance scores to their drivers (Helling, 2021; O’Donovan, 2017). In turn, these scores are used in various ways, such as inputs in determining job allocation. It is easy to see why drivers take such scores seriously – their very earnings and jobs depend on them (Cook, 2015; O’Donovan, 2017).
This article discusses what the author refers to as the ‘panoptic mode of automation.’ The panopticon concept was introduced in 1791 by English reformer Jeremy Bentham. It was later developed by French philosopher Michel Foucault in Discipline and punish: The birth of the prison. Generally, panopticism refers to a form of internal surveillance an individual exercises on themselves in response to the awareness of being monitored by an invisible watcher. Such panoptic power causes an individual to modify his behavior to conform with what he deems to be the watcher’s expectations (Foucault, 1977). In the panoptic mode of automation, drivers’ behavior is turned into machine-readable data, which is ultimately used to train machine learning algorithms to operate automated systems. To what extent is panoptic power baked into the Uber and Lyft platforms, and what techniques are used to engender it? These questions can, in part, be answered by looking more closely at the operational logics of the Uber and Lyft platforms. The following section attempts this task by critically examining the patents of these two companies.
Critical patent analysis
A patent is a legal and monopolistic right granted by the state to an inventor in exchange for disclosing an invention to the public. Technology and business practitioners have long used patent documents to track technological trends, identify innovation opportunities, and many other uses. In the social sciences, patents have also been used to examine social issues embedded in technology. For example, Delfanti and Frey (2021) used patent data to expose how Amazon uses its workers to automate its future warehouses. Shapiro (2020) also used patent data to examine how the ‘urban technocratic class’ patent smart cities and the lived experiences of the urban population. This article similarly uses patent data to explore a social issue baked into technology.
The analysis of Uber and Lyft’s patents will proceed in two stages. First, a brief overview of these companies’ patenting activity is provided. Second, a micro-level analysis of two patents will be employed to delve deeper into specific technologies.
Figure 1 shows Uber and Lyft’s patenting activities in the last few years. Due to a delay in patent publication, the years 2020 and 2021 are omitted from figure 1. However, what can be seen is that Uber and Lyft are very active in the patent space. An increase in patenting activity generally suggests growing innovation in specific technologies. Using patent classification codes, which are codes used to classify technologies, it is possible to identify the particular technologies most actively patented.
Figure 1. Uber and Lyft’s patenting activity (N = 2875).
Figure 2 shows Uber and Lyft’s top 10 most actively patented technologies. Being transport service providers, it is no surprise that technologies related to navigation (G01C21/00), control of position (G05D1/00), and traffic control systems for road vehicles (G08G1/00) are among the most actively patented. Uber and Lyft are also actively patenting technologies related to the retrieval of information (G06F16/00), processing of data so that it is readable by a computer (G06F3/00), and machine learning (G06N20). The key takeaway from the information presented in figure 2 is that data extraction, transformation, and utilization are key logics of the Uber and Lyft platforms.
Figure 2. Top technologies patented by Uber and Lyft.
To illustrate how these technologies of data extraction, transformation, and utilization work, two examples of patents by Uber and Lyft are provided. The first patent by Lyft titled Systems and methods for detecting and recording anomalous vehicle events (Clément, Lurie & Omari, 2020) is for an invention that uses a plethora of sensors to capture data associated with a vehicle and its drivers. Sensors include but are not limited to speed sensors, steering angle sensors, braking pressure sensors, GPS, and driver-facing cameras. These sensors capture a range of data, such as a driver’s eye or head movements in response to an abnormal event. The contextual data captured through these sensors is stored on the vehicle’s local computer or the cloud. The patent further states that such data may be used to train machine learning algorithms to drive autonomous vehicles. This patent makes it clear that drivers are a crucial component in the push for driverless vehicles. This observation is similar to that made by Delfanti and Frey (2021). They observed that Amazon’s workers are not about to disappear from its warehouses, despite the push for automation at such facilities. Like Amazon, Uber and Lyft use their workers as data generators to help build future automated systems.
The second patent by Uber, titled Providing user feedback for transport services through use of mobile devices (Radhakrishnan, Camp, Salazar & Kalanick, 2013), is for providing passenger feedback following an Uber ride. Figure 3 shows the user interface for passengers to give a quantitative score for a recently completed ride. There is also a comment section where passengers can comment on their experience.
Figure 3. A user interface to provide driver rating and comments on Uber (Radhakrishnan, Camp, Salazar & Kalanick, 2013).
Figure 4 shows a second user interface screen where passengers can provide more details about their experience. On this screen, passengers can specify which aspects of the ride experience were pleasing or unsatisfactory. Section [0067] of the patent explains how such ratings may be used:
The transport service may prioritize (or emphasize) the selection of drivers with good ratings. For example, the invite may first be sent to proximate drivers with [the] highest ratings, then proximate drivers with middle tier ratings. Still further, some embodiments provide the user with the ability to reject the driver based on, for example, the driver’s rating information. (Radhakrishnan, Camp, Salazar & Kalanick, 2013, p. 7)
This patent provides a good example of how surveillance is built into digital platforms such as Uber and Lyft and how these platforms outsource quality control to users (see Van Doorn, 2017).
Figure 4. A user interface to provide driver feedback on Uber (Radhakrishnan, Camp, Salazar & Kalanick, 2013).
Platform panopticism
In 1791, English legislative reformer, Jeremy Bentham, published a proposal of what he believed was an innovative prison model – the panopticon (Strub, 1989). The panoptic prison’s major features were a circular array of prison cells with a guard tower in the middle. The guard in the tower could observe any inmate at any time without being seen by the prisoners. Aware of being watched, prisoners would self-discipline and behave in a manner that they thought was promoted by the watchers. In Discipline and Punish, Foucault explains how panoptic disciplinary power works.
He who is subjected to a field of visibility, and who knows it, assumes responsibility for the constraints of power; he makes them play spontaneously upon himself; he inscribes in himself the power relation in which he simultaneously plays both roles; he becomes the principle of his own subjection (Foucault, 1977 p. 202-203).
Bentham thought that such a system could be applied in any establishment where people must be kept under inspection. These include houses of correction, workhouses, manufactories, mad-houses, hospitals, and schools (Bentham, 1791).
The ideas of platform surveillance, which are at the heart of Uber and Lyft platforms, are quite similar to Jeremy Bentham’s “ideal prison.” Although the ‘power of the gaze’ appears to have been largely superseded by the ‘power of computation’ in the case of platforms, the awareness of perpetual visibility remains. According to the patents shown in this study, drivers may be constantly monitored through various sensors on their vehicles, mobile phones, and passengers. The vehicles used by Uber and Lyft are in essence “panopticism on wheels.” The panopticon, as Sheridan (2016) correctly observes, “has been recast in security cameras and algorithms, police presence, and data trawlers” (p.3). As a result, in today’s digital world, where visibility is more enhanced, the panopticon seems more relevant than in the nineteenth century (Manokha, 2018).
To think of platforms as digital panopticons is to acknowledge how platform workers are watched and how that monitoring molds their behavior. Even in its potential form, surveillance can influence worker behavior in favor of platform owners. Panopticism on platforms like Uber and Lyft has another purpose in addition to managing and governing drivers: it optimizes the platform toward increasing levels of automation. It was observed from the patents how data from drivers are utilized for training machine learning models to operate autonomous vehicles. This human-machine interface is what the author refers to as the ‘panoptic mode of automation’ or ‘panoptic-enable automation.’
Platform panopticism seems to be a part of what Zuboff (2019) has referred to as ‘surveillance capitalism.’ While Zuboff’s (2019) notion of surveillance capitalism is an economic model centered on the extraction and commercialization of user data, platform panopticism refers to the strategic engineering of workers’ behavior through the operationalization of surveillance. Uber and Lyft are thus a part of the surveillance capitalists described by Zuboff (2019) in her book The Age of Surveillance Capitalism.
Conclusion
This article aimed to show how panopticism is built into the Uber and Lyft platforms. Through a critical analysis of patents by these companies, the article showed how surveillance forms part of the push for an automated future. Such automation will be achieved by extracting significant amounts of data on drivers provided by a plethora of sensors and passenger feedback. This human-machine interface was referred to as the ‘panoptic mode of automation’ or ‘panoptic-enabled automation.’
Through the panoptic mode of automation, platform owners may study drivers and convert every behavior into machine-readable signals using algorithms and a slew of sensors. Drivers who are aware that they are being observed act ‘properly,’ reinforcing the platform’s rules while also generating plenty of data for future automated systems. This does not suggest that drivers have no power or operate in the same way on platforms. Indeed, some research demonstrates that learning the platform’s rules helps drivers build resistance (Allen-Robertson, 2017). There is still much that remains unsaid about the panoptic mode of automation. Perhaps future studies can adopt a combined approach where patent data is supplemented with stories from the streets. Such an approach will offer a richer analysis of the working of platforms.
Bibliography
Allen-Robertson, J. (2017). The Uber Game: Exploring Algorithmic Management and Resistance. AoIR Selected Papers of Internet Research.
Bentham, J. (1791). Panopticon or the inspection house (Vol. 2).
Clément, R., Lurie, H. R., & Omari, S. (2020). U.S. Patent Application No. 16/107,986.
Cook, J. (2015). Uber’s internal charts show how its driver-rating system actually works. Retrieved from https://www.businessinsider.com/leaked-charts-show-how-ubers-driver-rating-system-works-2015-2
Delfanti, A., & Frey, B. (2021). Humanly extended automation or the future of work seen through Amazon patents. Science, Technology, & Human Values, 46(3), 655-682.
Foucault, M. (1977). Discipline and punish: The birth of the prison. London: Penguin Books.
Helling, B. (2021). Understanding the Lyft Driver Rating System. Retrieved from https://www.ridester.com/lyft-driver-rating/
Korosec, K. (2020). Lyft is using data from its rideshare drivers to develop self-driving cars. Retrieved from https://techcrunch.com/2020/06/23/lyft-is-using-data-from-its-ride-share-drivers-to-develop-self-driving-cars/
Manokha, I. (2018). Surveillance, panopticism, and self-discipline in the digital age. Surveillance & Society, 16, 219-237.
O’Donovan, C. (2017). That Four-Star Rating You Left Could Cost Your Uber Driver Her Job. Retrieved from https://www.buzzfeednews.com/article/carolineodonovan/the-fault-in-five-stars
Radhakrishnan, M., Camp, G., Salazar, O., & Kalanick, T. C. (2013). U.S. Patent Application No. 13/837,592.
Rosenblat, A., & Stark, L. (2016). Algorithmic labor and information asymmetries: A case study of Uber’s drivers. International journal of communication, 10, 27.
Sadowski, J. (2019). When data is capital: Datafication, accumulation, and extraction. Big Data & Society, 6(1), 2053951718820549.
Shapiro, A. (2020). ‘Embodiments of the invention’: Patents and urban diagrammatics in the smart city. Convergence, 26(4), 751-774.
Sheridan, C. (2016). Foucault, power and the modern panopticon. Senior Theses. Hartford: Trinity College.
Strub, H. (1989). The theory of panoptical control: Bentham’s panopticon and Orwell’s Nineteen Eighty‐Four. Journal of the History of the Behavioral Sciences, 25, 40-59.
Van Doorn, N. (2017). Platform labor: on the gendered and racialized exploitation of low-income service work in the ‘on-demand’economy. Information, Communication & Society, 20, 898-914.
Zuboff, S. (2019). The age of surveillance capitalism: The fight for a human future at the new frontier of power: Barack Obama’s books of 2019. Profile books.
[PDF]