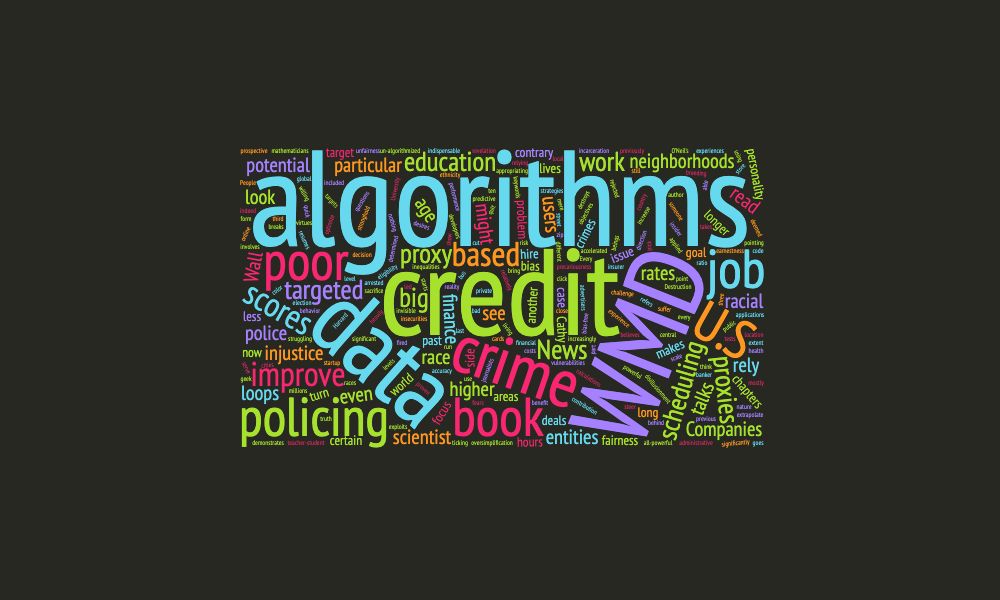
Weapons of Math Destruction: How Big Data Increases Inequality and Threatens Democracy
Book review by Lungani Hlongwa
Abstract: Algorithms are almost everywhere. They decide who gets the mortgage loan and who doesn’t; who gets hired and gets fired; who gets released from prison, and who should serve longer. Today, it is tough to imagine a sphere that has not embraced algorithmic power. These mathematical entities are increasingly taking over areas previously reserved for human decision-making. Since algorithms make decisions based on big data, they are mostly considered bias-free and objective in their decision-making. However, a growing number of scholars and mathematicians are taking a second look at algorithmic power. Many are pointing out some of the inequalities built into these seemingly all-powerful entities. Cathy O, Neil’s book is one such contribution to the literature. The title of her book sums up her view on algorithms. In her book, O’Neil shows how algorithms breed inequality while threatening democracy.
Keywords: algorithms, big data, inequality, democracy, justice, power
Header image created by author.
At a time when decision making is no longer the province of human beings, O’Neil’s book is a much-needed read for those who want to understand the power of algorithms. Cathy O’Neil is an American mathematician and author who obtained her Ph.D. in mathematics from Harvard University. With her experience in Wall Street in finance, few can provide an inside look at the work that algorithms do in a way that O’Neil does. Her book is divided into ten chapters that take the reader through her journey from being a math geek to an insider who witnessed the “dark side” of algorithmic power.
In the first chapter titled “BOMB PARTS: What Is a Model?” O’Neil breaks down the algorithmic model. She argues that mathematical models are prone to mistakes since they are, by nature, an oversimplification of reality. This is true for algorithms as they rely on data to extrapolate truth. O’Neil then makes an example with incarceration rates to show how bias can be built into algorithms. If models are trained to see certain races as more likely to commit crimes, then surely there will be more incarcerations in those racial groups. O’Neil argues that we have not reduced bias with these mathematical models but camouflaged it with technology. For O’Neil, there are three elements of weapons of math destruction, namely, Opacity, Scale, and Damage.
In chapter 2, O’Neil provides a historical account of her experiences as a quant in Wall Street. She discusses how she came to see the people behind the algorithms who wield numbers and equations as weapons. This revelation prompted her to leave Wall Street to join a startup as a data scientist. However, not long after she began working as a data scientist, she realized that finance and big data had a lot in common. Both relied heavily on imperfect models, and both were out of touch with “real people.” O’Neil refers to these imperfect models as weapons of math destruction (WMD). With big data, her main concern was how people were being turned into data. O’Neil saw how inequality was rising and how mathematics was being misused. All this accelerated her disillusionment, which led her to quit her job as a data scientist to investigate issues with more earnestness.
In the third chapter of her book, O’Neil discusses WMD in the education system. She goes into detail explaining how journalists from the U.S. News created algorithms to rank universities. U.S. News used proxies like SAT scores, acceptance rates, and teacher-student ratio to determine the universities’ ranking. In turn, universities attempted to improve their rankings by ticking all the boxes deemed relevant by U.S. News. O’Neil argues that since proxies are easy to manipulate, many universities could improve their rankings by falsifying their data. Ultimately, O’Neil contends that a ranking system like that of U.S. News does nothing for the education system. On the contrary, it destroys the education system by reducing university performance to mere data.
In chapter 4, O’Neil takes the reader deeper into the world of targeted advertising. Companies benefit greatly from users online by appropriating their data. Every like, click, or preference provides an opportunity for companies to get to know our habits, fears, and desires. O’Neil provides an example of how immigrant students come to the U.S., believing that private universities are better than public ones. O’Neil discusses how algorithms target specific groups of people based on their vulnerabilities or insecurities. The scale of targeted advertising is massive. Advertisers can target millions of people every day with their products and services. Advertisers are also driven to learn about those advertising strategies that are most likely to lead to a purchase. Was it the ad at the bus stop, the ad on Facebook or YouTube, or the one at the local grocery store? With such information in hand, advertisers can optimize their campaigns and improve overall purchases. This is the goal of targeted advertising, and WMD are central to this goal.
Chapter 5 was a rather interesting read as it deals with the issue of justice. In this chapter, O’Neil talks about how algorithms are used in the justice system in the U.S. She argues that developers of crime predicting algorithms are desperate to include an ever-larger number of proxies into their models to improve their accuracy. However, O’Neil contends that these models have their own virtues, even though they are often presented as blind to race and ethnicity. She makes an example with PredPol, a crime predicting model widely used by police departments in the U.S. According to the promoters of PredPol, the software is blind to race and targets geography instead. However, O’Neil points out that in racially segregated cities, geography can be a proxy for race. In other words, even if the algorithms are color blind, the result would be a higher number of incarcerations of particular racial groups.
O’Neil points to another problem with relying on geography as a proxy for crime. Once algorithms have determined that certain areas need more policing, the policing itself creates new data. In other words, more policing justifies the need for more policing. Such feedback loops are very common in WMD. O’Neil then wonders how such a crime predictive software might be applied in finance. She believes that many people would be arrested for financial crimes. However, she argues that the police are not trained to deal with white-collar crime. On the contrary, they are trained to tackle crime on the street and in impoverished neighborhoods. According to O’Neil, PredPol is a WMD in that it empowers the police to focus on impoverished populations. The result is that poverty and crime are collapsed onto one another. She argues that fairness is not included in the calculations of WMD. The case of uneven policing demonstrates this phenomenon very well. O’Neil wonders whether we would be willing to sacrifice a few algorithmic efficiencies for the sake of fairness.
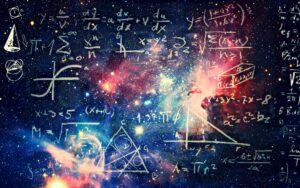
In chapter 6, O’Neil discusses how algorithms are used in the hiring process. Many HR departments have turned to algorithms to make the right hire and to reject those considered unsuitable for the job. For most HR departments, using algorithms to surf out potential employees is a strategy to cut administrative costs. O’Neil argues that only those with the resources and best resumes make it past the algorithms. For this reason, many potential job seekers now focus on crafting the perfect resume to make it through algorithmic screening. This often involves including specific keywords that are considered indispensable for particular applications. O’Neil also discusses how personality tests are used to determine the right candidate for the job. She argues that such approaches have not been proven to help companies hire the right candidate. Instead, many potential job seekers do not make it past the personality test and algorithms. Many are then blocked out of the job market.
Chapter 7 discusses the precariousness of work in the age of WMD. O’Neil raises the issue of scheduling for employees of companies like Starbuck, McDonald’s, and Walmart. Many employees in these companies work long hours and face increased levels of stress. O’Neil is quick to point out scheduling software as WMD. The algorithms that run such software sometimes schedule the same employee to open and close the shop, leaving him with only a few hours of rest. This practice has become known as “clopening,” and employees suffer significantly from it. Scheduling software is, according to O’Neil, a WMD because it is massive, and it exploits people already struggling to make a living. Scheduling algorithms are also very opaque. Workers sometimes have no idea when they will be required to work. They are summoned by invisible algorithms that wield ever-more power over their lives. Like other WMD, O’Neil argues that scheduling software also has significant feedback loops. It is almost as if they were designed to keep the poor in a state of perpetual poverty. The root of the problem, according to O’Neil, is the modeler’s objectives. Companies rely on scheduling software to improve efficiency and to increase profit, not to for justice.
In chapter 8, O’Neil talks about how WMD lock some people out of credit. Using credit scores, algorithms can determine who gets credit and who doesn’t. Credit card companies also rely on credit scores to determine one’s eligibility for credit cards. Such credit scores are primarily based upon one’s previous credit behavior. WMD in the credit system use a range of proxies to determine credit eligibility and how much people are eligible for. One proxy that is particularly troubling for O’Neil is the “zip code.” As with other WMD, geography can be a proxy for racial segregation. People from affluent neighborhoods are more likely to get more credit, while those from poor settlements either get less credit with higher interest rates or are rejected altogether. O’Neil also notes the feedback loop that exists in WMD in the credit system. Sometimes, prospective employees will be denied a job based on their credit record. If one is refused a loan based on one’s credit record, that record will most likely worsen, which in turn reduces the chances of being employed. Such feedback loops are, according to O’Neil, ubiquitous in WMD.
Chapter 9 deals with insurance. Some people are denied insurance because they come from poor neighborhoods. They are considered high risk and most likely to default on their payments. Denying someone insurance coverage because of their location is known as “redlining.” According to O’Neil, redlining is still prevalent today, even though in a more subtle form. Redlining has now become the province of algorithms. It is the algorithms that decide who gets the loan and not the banker himself. O’Neil also argues that insurance works against the poor. Sometimes insurance companies would look at one’s credit to determine the level of coverage. Those with higher credit often receive less coverage from the insurer. O’Neil wonders whether health scores might be used one day to determine who gets hired in the age of ubiquitous computing. Such a future is indeed a dystopian one where inequality and unfairness will be the order of the day.
In the last chapter, O’Neil talks about how citizens might be targeted. She starts by discussing the power that Facebook has with its ability to tweak what users see on their newsfeed. She argues that Facebook is not the only company with so much power. Companies like Microsoft, Google, Version, and AT&T also hold vast amounts of information on their users. Facebook, according to O’Neil, is not only massive but also powerful and opaque. O’Neil notes how Facebook could steer voters in a particular direction and influence the politics of a country, as was the case during the 2016 election in the U.S.
Commentary
This book provides essential lessons on how to think about algorithmic power. It questions the injustice and inequality propagated by these seemingly omniscient entities that control an ever-larger part of our lives. The various chapters in this book touch on different domains that have become breeding grounds for algorithms. These domains include the education system, advertising, job markets, the credit system, and insurance. It is hard to imagine a sphere that has not embraced algorithms to some extent. In the age of growing injustice and inequality, Weapons of Math Destruction is a good read for those interested in seeing the “dark side” of algorithms. Countries where algorithmic power has not taken a stronghold, particularly those in the global South, have much to learn from those where algorithmic inequality and injustice are the order of the day. There is both good and bad to algorithms, and O’Neil is also cognizant of this. A challenge then for the relatively “un-algorithmized” world is to pick and choose the good that algorithms can bring.
REFERENCES
O’Neil, C. (2016). Weapons of math destruction: How big data increases inequality and threatens democracy. Broadway Books.